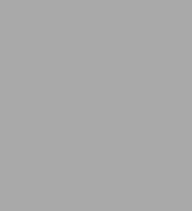
Applied Regression Analysis and Generalized Linear Models
816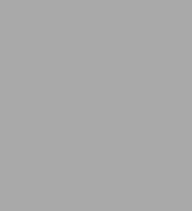
Applied Regression Analysis and Generalized Linear Models
816eBookThird Edition (Third Edition)
Related collections and offers
Overview
Accompanying website resources containing all answers to the end-of-chapter exercises. Answers to odd-numbered questions, as well as datasets and other student resources are available on the author′s website.
NEW! Bonus chapter on Bayesian Estimation of Regression Models also available at the author′s website.
Product Details
ISBN-13: | 9781483321318 |
---|---|
Publisher: | SAGE Publications |
Publication date: | 03/18/2015 |
Sold by: | Barnes & Noble |
Format: | eBook |
Pages: | 816 |
File size: | 41 MB |
Note: | This product may take a few minutes to download. |
About the Author
John Fox received a BA from the City College of New York and a PhD from the University of Michigan, both in Sociology. He is Professor Emeritus of Sociology at McMaster University in Hamilton, Ontario, Canada, where he was previously the Senator William McMaster Professor of Social Statistics. Prior to coming to McMaster, he was Professor of Sociology, Professor of Mathematics and Statistics, and Coordinator of the Statistical Consulting Service at York University in Toronto. Professor Fox is the author of many articles and books on applied statistics, including \emph{Applied Regression Analysis and Generalized Linear Models, Third Edition} (Sage, 2016). He is an elected member of the R Foundation, an associate editor of the Journal of Statistical Software, a prior editor of R News and its successor the R Journal, and a prior editor of the Sage Quantitative Applications in the Social Sciences monograph series.
Table of Contents
PrefaceAbout the Author
1. Statistical Models and Social Science
1.1 Statistical Models and Social Reality
1.2 Observation and Experiment
1.3 Populations and Samples
I. DATA CRAFT
2. What Is Regression Analysis?
2.1 Preliminaries
2.2 Naive Nonparametric Regression
2.3 Local Averaging
3. Examining Data
3.1 Univariate Displays
3.2 Plotting Bivariate Data
3.3 Plotting Multivariate Data
4. Transforming Data
4.1 The Family of Powers and Roots
4.2 Transforming Skewness
4.3 Transforming Nonlinearity
4.4 Transforming Nonconstant Spread
4.5 Transforming Proportions
4.6 Estimating Transformations as Parameters*
II. LINEAR MODELS AND LEAST SQUARES
5. Linear Least-Squares Regression
5.1 Simple Regression
5.2 Multiple Regression
6. Statistical Inference for Regression
6.1 Simple Regression
6.2 Multiple Regression
6.3 Empirical Versus Structural Relations
6.4 Measurement Error in Explanatory Variables*
7. Dummy-Variable Regression
7.1 A Dichotomous Factor
7.2 Polytomous Factors
7.3 Modeling Interactions
8. Analysis of Variance
8.1 One-Way Analysis of Variance
8.2 Two-Way Analysis of Variance
8.3 Higher-Way Analysis of Variance
8.4 Analysis of Covariance
8.5 Linear Contrasts of Means
9. Statistical Theory for Linear Models*
9.1 Linear Models in Matrix Form
9.2 Least-Squares Fit
9.3 Properties of the Least-Squares Estimator
9.4 Statistical Inference for Linear Models
9.5 Multivariate Linear Models
9.6 Random Regressors
9.7 Specification Error
9.8 Instrumental Variables and Two-Stage Least Squares
10. The Vector Geometry of Linear Models*
10.1 Simple Regression
10.2 Multiple Regression
10.3 Estimating the Error Variance
10.4 Analysis-of-Variance Models
III. LINEAR-MODEL DIAGNOSTICS
11. Unusual and Influential Data
11.1 Outliers, Leverage, and Influence
11.2 Assessing Leverage: Hat-Values
11.3 Detecting Outliers: Studentized Residuals
11.4 Measuring Influence
11.5 Numerical Cutoffs for Diagnostic Statistics
11.6 Joint Influence
11.7 Should Unusual Data Be Discarded?
11.8 Some Statistical Details*
12. Non-Normality, Nonconstant Error Variance, Nonlinearity
12.1 Non-Normally Distributed Errors
12.2 Nonconstant Error Variance
12.3 Nonlinearity
12.4 Discrete Data
12.5 Maximum-Likelihood Methods*
12.6 Structural Dimension
13. Collinearity and Its Purported Remedies
13.1 Detecting Collinearity
13.2 Coping With Collinearity: No Quick Fix
IV. GENERALIZED LINEAR MODELS
14. Logit and Probit Models for Categorical Response Variables
14.1 Models for Dichotomous Data
14.2 Models for Polytomous Data
14.3 Discrete Explanatory Variables and Contingency Tables
15. Generalized Linear Models
15.1 The Structure of Generalized Linear Models
15.2 Generalized Linear Models for Counts
15.3 Statistical Theory for Generalized Linear Models*
15.4 Diagnostics for Generalized Linear Models
15.5 Analyzing Data From Complex Sample Surveys
V. EXTENDING LINEAR AND GENERALIZED LINEAR MODELS
16. Time-Series Regression and Generalized Leasr Squares*
16.1 Generalized Least-Squares Estimation
16.2 Serially Correlated Errors
16.3 GLS Estimation With Autocorrelated Errors
16.4 Correcting OLS Inference for Autocorrelated Errors
16.5 Diagnosing Serially Correlated Errors
16.6 Concluding Remarks
17. Nonlinear Regression
17.1 Polynomial Regression
17.2 Piece-wise Polynomials and Regression Splines
17.3 Transformable Nonlinearity
17.4 Nonlinear Least Squares*
18. Nonparametric Regression
18.1 Nonparametric Simple Regression: Scatterplot Smoothing
18.2 Nonparametric Multiple Regression
18.3 Generalized Nonparametric Regression
19. Robust Regression*
19.1 M Estimation
19.2 Bounded-Influence Regression
19.3 Quantile Regression
19.4 Robust Estimation of Generalized Linear Models
19.5 Concluding Remarks
20. Missing Data in Regression Models
20.1 Missing Data Basics
20.2 Traditional Approaches to Missing Data
20.3 Maximum-Likelihood Estimation for Data Missing at Random*
20.4 Bayesian Multiple Imputation
20.5 Selection Bias and Censoring
21. Bootstrapping Regression Models
21.1 Bootstrapping Basics
21.2 Bootstrap Confidence Intervals
21.3 Bootstrapping Regression Models
21.4 Bootstrap Hypothesis Tests*
21.5 Bootstrapping Complex Sampling Designs
21.6 Concluding Remarks
22. Model Selection, Averaging, and Validation
22.1 Model Selection
22.2 Model Averaging*
22.3 Model Validation
VI. MIXED-EFFECT MODELS
23. Linear Mixed-Effects Models for Hierarchical and Longitudinal Data
23.1 Hierarchical and Longitudinal Data
23.2 The Linear Mixed-Effects Model
23.3 Modeling Hierarchical Data
23.4 Modeling Longitudinal Data
23.5 Wald Tests for Fixed Effects
23.6 Likelihood-Ratio Tests of Variance and Covariance Components
23.7 Centering Explanatory Variables, Contextual Effects, and Fixed-Effects Models
23.8 BLUPs
23.9 Statistical Details*
24. Generalized Linear and Nonlinear Mixed-Effects Models
24.1 Generalized Linear Mixed Models
24.2 Nonlinear Mixed Models
Appendix A
References
Author Index
Subject Index
Data Set Index