Contrasting Responses of Rhizosphere Bacterial, Fungal, Protist, and Nematode Communities to Nitrogen Fertilization and Crop Genotype in Field Grown Oilseed Rape (Brassica napus)
- 1School of Life Sciences, University of Warwick, Coventry, United Kingdom
- 2School of Life and Medical Sciences, University of Hertfordshire, Hatfield, United Kingdom
- 3Rothamsted Research, Harpenden, United Kingdom
The rhizosphere microbiome is considered to play a key role in determining crop health. However, current understanding of the factors which shape assembly and composition of the microbiome is heavily biased toward bacterial communities, and the relevance for other microbial groups is unclear. Furthermore, community assembly is determined by a variety of factors, including host genotype, environment and agricultural management practices, and their relative importance and interactions remain to be elucidated. We investigated the impact of nitrogen fertilization on rhizosphere bacterial, fungal, nematode and protist communities of 10 contrasting oilseed rape genotypes in a field experiment. We found significant differences in the composition of bacteria, fungi, protist and nematode communities between the rhizosphere and bulk soil. Nitrogen application had a significant but weak effect on fungal, bacterial, and protist community composition, and this was associated with increased relative abundance of a complex of fungal pathogens in the rhizosphere and soil, including Mycosphaerella sp. and Leptosphaeria sp. Network analysis showed that nitrogen application had different effects on microbial community connectivity in the soil and rhizosphere. Crop genotype significantly affected fungal community composition, with evidence for a degree of genotype specificity for a number of pathogens, including L. maculans, Alternaria sp., Pyrenopeziza brassicae, Olpidium brassicae, and L. biglobosa, and also potentially beneficial Heliotales root endophytes. Crop genotype had no significant effect on assembly of bacteria, protist or nematode communities. There was no relationship between genetic distance of crop genotypes and the extent of dissimilarity of rhizosphere microbial communities. Field disease assessment confirmed infection of crops by Leptosphaeria sp., P. brassicae, and Alternaria sp., indicating that rhizosphere microbiome sequencing was an effective indicator of plant health. We conclude that under field conditions soil and rhizosphere nutrient stoichiometry and crop genotype are key factors determining crop health by influencing the infection of roots by pathogenic and mutualistic fungal communities, and the connectivity and stability of rhizosphere microbiome interaction networks.
Introduction
Plant roots live in intimate association with diverse microbial communities which can have major impacts on their health, growth, and productivity. Plants secrete up to 20% of the carbon they fix into the soil as exudates, particularly in the form of sugars and organic acids, and this drives the selective growth of microbes in the soil immediately adjacent to roots, and the recruitment of microbes onto the root surface and within the root itself (Morgan et al., 2005). The microbiota residing in roots and the soil surrounding the roots constitutes the rhizosphere microbiome, which can influence the plant through diverse direct and indirect interaction pathways (Hunter et al., 2014; Hilton et al., 2021).
Root symbionts such as arbuscular fungi have potential to provide their host with access to nutrients and water in exchange for carbon, while pathogens within the root zone can cause disease. Additionally, free-living rhizosphere microbes can indirectly exert beneficial and detrimental impacts on the plant, e.g., by stimulating or suppressing the growth of pathogens and symbionts, and through nutrient mobilization-immobilization processes (Morgan et al., 2005). There is considerable interest in understanding rhizosphere microbiome assembly processes in order to unravel what constitutes a microbiome which benefits the host, and the contributors to microbiome dysbiosis, which results in harm to the host (Tabrett and Horton, 2020; Hilton et al., 2021).
The composition of the rhizosphere microbiome is determined by complex interactions between the soil, the plant and the environment which determine recruitment of biota from the soil (Jones et al., 2004). The plant itself plays a major role in selecting rhizosphere microbiota, and microbiome composition differs between plant species, reflecting quantitative and qualitative differences in exudation, plant nutrient and water uptake dynamics, and root characteristics, such as the presence of root hairs. Effects of the plant on the rhizosphere microbiome are further modulated by factors which impact exudation, such as plant development status, soil nutrient characteristics and light intensity (Jones et al., 2004; Bulgarelli et al., 2015).
It is also firmly established that plant intraspecific genetic variation can influence microbiome composition. As a result, there is considerable interest in using breeding to engineer the rhizosphere microbiome, harnessing functional traits conducive for sustainable agriculture, such as disease suppression and nutrient mobilization (Hunter et al., 2014). While crop cultivar differences in microbiomes have been noted for a range of field grown crops, including cotton (Wei et al., 2019), oilseed rape (Taye et al., 2020), soybean (Zhong et al., 2019), and wheat (Mahoney et al., 2017), the focus has been on bacterial communities with a striking absence of data on microbial eukaryotes. Fungi, protists, and nematodes can all directly affect plant health through pathogenic and parasitic interactions (Hunter et al., 2014), while arbuscular fungi are among the best documented beneficial symbionts (Gosling et al., 2006). Furthermore, grazing by protists is postulated to play a major role in determining the structure of both bacterial and fungal communities in the rhizosphere (Gao et al., 2019).
Microbiome assembly under field conditions is under the influence of a wide range of variables including fertilizer and fungicide application, and the role of genotype in determining microbiome composition, relative to other factors needs to be understood if it is to be utilized as an effective crop management tool (Rathore et al., 2017). For example, evidence from long term field experiments suggests that fertilization may exert a greater impact on the rhizosphere bacterial community than plant species (Semenov et al., 2020).
Globally, oilseed rape (OSR) is the third largest source of vegetable oil, with 70 million tons produced worldwide each year, and is the third most cultivated crop in the UK by area (FAOstat, 2018; DEFRA: Agriculture in the United Kingdom., 2019). While there is considerable understanding of microbiome communities in the brassicaceous model Arabidopsis thaliana, the assembly and function of the microbiome of OSR has received little attention. However, there is evidence for OSR cultivar differences in rhizosphere bacterial community composition (Marschner et al., 2007), which persist under field conditions (Taye et al., 2020), and furthermore, OSR cultivars can show differential responses to the fungal pathogen Verticillium longisporum, potentially associated with differences in antagonistic endophytic bacterial communities (Glaeser et al., 2019).
In this study we investigated the influence of cultivar and nitrogen fertilizer application on the assembly of the OSR rhizosphere microbiome. Ten OSR cultivars were grown in the field with and without conventional application of inorganic nitrogen fertilizer, and the diversity, composition and connectivity of soil and rhizosphere bacteria, fungi, protists and nematode communities was investigated.
Materials and Methods
Location, Experimental Design, and Sampling Procedure
The field experiment was conducted at Rothamsted Research, Hertfordshire, UK (51.803896°N−0.362505°E) on a flinty clay loam soil (27% clay, pH 7.3, total carbon 2.9% and total nitrogen (N) 0.24%). Ten different B. napus genotypes [Canard DH13 (Canard), Couve Nabica DH2 (Couve Nabica), Darmor, Ningyou 7, Rocket × Lizard DH Line (Rocket), Tapidor, Temple, Victor, York, and Yudal; Table 1] were selected to encompass the genetic variability available within OSR and its wider breeding material. Several genotypes possessed known resistance/susceptibility to OSR fungal pathogens (Table 1). Each genotype was grown in four 10 × 3 m plots (60 seeds/m2) over the 2013–2014 growing season, two with the conventional recommended ammonium nitrate fertilizer rate of ~210 kg N ha−1 (30 kg N ha−1 applied on 17/09/2013, and 174 N ha−1 applied in 05/03/2014), and two with no nitrogen application, and representing high and low nitrogen treatments, respectively. Crops were drilled on 31/08/2013 and received herbicides and molluscicides according to local best agronomic practices. No fungicides were applied.
Soil and rhizosphere samples were collected immediately post-flowering on 27/04/2014, during the pod development stage. Four plants were dug up equidistant to each other, from 1 m inside each plot, and pooled to comprise one sample from each plot. Roots were shaken free of loose soil and fine roots were cut to ~5 mm sections. Fine roots together with adhering soil were designated as rhizosphere samples. Bulk soil was collected adjacent to each plant to a depth of 12 cm, the top 2 cm discarded, and cores combined for each plot before being sieved <4 mm. This process gave four independent root and soil replicates for each crop genotype; two under each nitrogen treatment. DNA was extracted from 0.5 g of rhizosphere and bulk soil samples using the FastDNA® SPIN Kit for Soil, according to the manufacturer's instructions (MP Biomedicals, Santa Ana, USA). DNA was quantified using the Qubit 2.0 Fluorometer (Life Technologies, USA) with the broad range DNA assay and diluted to 5 ng μl−1.
Genetic Similarity of Brassica Genotypes
A collection of 189 accessions representing the diversity within the Brassica napus species gene pool (known as the Brassica napus diversity fixed foundation set; BnDFFS) developed by the UK Defra-funded Oilseed Rape Genetic Improvement Network (OREGIN; https://www.herts.ac.uk/oregin/about-oregin) project were genotyped with the Illumina Brassica napus 60 K Infinium assay (performed by Trait Genetics, Gatersleben, Germany). Single nucleotide polymorphism (SNP) markers were retained for analysis of the 10 genotypes used in the current study where they were polymorphic with a minor allele frequency of at least 5%. The vegan package (Oksanen et al., 2018) was used to determine Euclidean distance for B. napus SNP data. Hierarchical clustering of the Euclidian distance of genotype SNP data was used to determine genetic similarity of the Brassica napus genotypes. Distance metrics were plotted using ggplot2 (Hamilton and Ferry, 2018). This demonstrated that Yudal, Ningyou 7, and York were genetically similar to each other but clearly distinct to the other genotypes, and that Couve Nabica and Canard clustered together, and were distinct from Victor, Temple, Rocket, and Darmor (Figure 1).
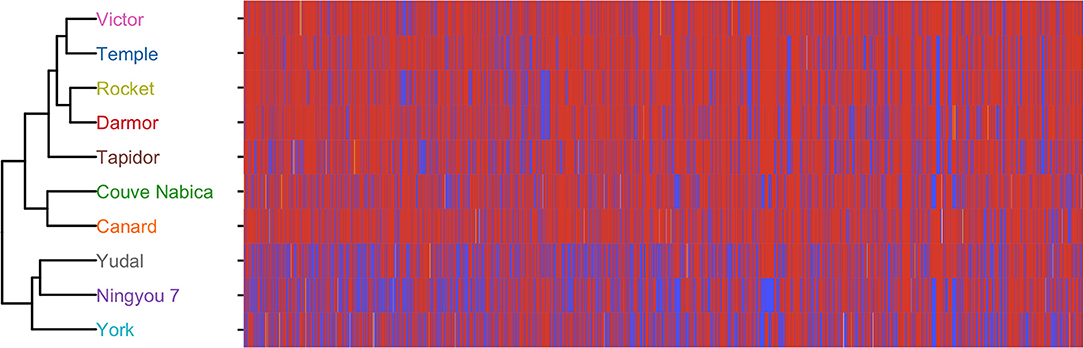
Figure 1. Dendrogram showing relatedness of the 10 Brassica genotypes used in this study based on 60 K single nucleic acid polymorphisms (SNP), and associated heatmap showing the SNP at each position. Red indicates presence of major alleles, blue minor alleles. Heterozygous calls are indicated in yellow.
Determination of Microbial Community Diversity and Structure
PCR amplification was performed on the extracted DNA using the 16S rRNA gene primers 515f and 806r to amplify bacterial sequences (Caporaso et al., 2012), Internal Transcribed Spacer (ITS) ITS3 and ITS4 primers for fungi (White et al., 1990), and the 18S rRNA gene primers Euk1391 and EukBr (Amaral-Zettler et al., 2009; Stoeck et al., 2010) for microbial eukaryotes. Primers were modified with Illumina Nextera Index Kit v2 adapters. PCRs were conducted using a Multigene Optimax thermal cycler (Labnet, USA), in a volume of 25 μl containing 15 ng DNA, Q5® Hot start High Fidelity 2× Master Mix (New England Biolabs) and 0.5 μM of each primer. Thermocycling included an initial denaturation at 98°C for 30s followed by 25 cycles (16S and 18S rRNA) or 30 cycles (ITS) of 98°C for 10s, 50/57°C (for 16S and 18S rRNA/ITS, respectively) for 15s and 72°C for 20s. The final extension was at 72°C for 5 min. PCR products were purified using the AMPure XP beads (Beckman Coulter, Germany) according to the manufacturer's instructions and the DNA concentrations were measured using the Qubit 2.0 Fluorometer (Life Technologies, USA) and diluted to 4 nM. The libraries were pooled and sequenced using the Illumina MiSeq Reagent Kit v300 for 2 × 300 bp sequencing.
Bioinformatic Analysis
Following sequencing, trimmomatic v0.35 (Bolger et al., 2014), was used to remove low-quality bases. Paired-ends reads were assembled by aligning the forward and reverse reads, primers were trimmed, and quality filtering was conducted with the setting (–fastq_maxee 0.5) using USEARCH and UPARSE software (Edgar, 2010, 2013).
Unique sequences were sorted by abundance and singletons were discarded. Chimeras were removed using the SILVA database as a reference (Quast et al., 2013), and sequences were clustered to Operational Taxonomic Units (OTUs) at 97% minimum identity threshold. Taxonomy was assigned using Quantitative Insights into Microbial Ecology (QIIME 1.8) (Caporaso et al., 2010) using the Greengenes reference database for 16S rRNA (McDonald et al., 2012), the Unite database for ITS (Koljalg et al., 2013) and the SILVA and PR2 databases for 18S rRNA (Guillou et al., 2013; Quast et al., 2013). The 16S rRNA OTU table was filtered to remove reads annotated as chloroplast, eukaryota, archaea, or mitochondria. Sequences from Archaeplastida, fungi, and metazoa were removed from the 18S rRNA dataset to leave predominantly single-celled eukaryotes, referred to hereafter as protists. The 18S rRNA dataset was also used to collect nematode sequences. Samples with below 3,000 reads were discarded. Post filtering, there were 4.9 million bacterial reads and 7647 OTUs, 1.3 million fungal reads across 961 OTUs, 127 K protist reads and 877 OTUs, and 21 K nematode reads across 85 OTUs (Supplementary Table 1). The datasets described in this paper are available in the NCBI sequence read archive under BioProject ID PRJNA666392. The sequences of OTUs highlighted in this study are deposited in the NCBI GenBank database under accession numbers MW051022-MW051025 (protists) and MW051900-MW051914 (fungi).
Microbial Community Analysis
Fisher's alpha diversity was calculated at the OTU level in R without normalization. For all other analyses, variation in sequencing depth was normalized using the median ratio of OTU counts with DESeq2 (Love et al., 2014). The vegan package was used to calculate Bray-Curtis distance for amplicon data and to perform PERMANOVA. Distance metrics were visualized using non-metric multidimensional scaling ordination, and plotted using ggplot2. Mantel tests were used to compare the Euclidian distance matrix of the B. napus genotypes, as determined using SNP data, with the Bray Curtis distance matrix of each microbial group in the rhizosphere.
Individual OTU contributions to the differences in overall community structure were calculated using similarity percentage (SIMPER). For multiple comparisons of genotype differences SIMPER was performed using PAST4 (Hammer et al., 2001). For pairwise comparisons between nitrogen treatments, the vegan implementation of SIMPER was used. Dufrene-Legendre indicator species analysis (Dufrêne and Legendre, 1997) was performed using the labdsv package in R to identify OTUs linked to either B. napus genotype or nitrogen treatment.
To investigate shifts in community connectivity due to the altered nitrogen inputs, inter-kingdom network analysis (Hilton et al., 2021) was performed using the top quartile of most abundant bacterial, protist, nematode and fungal OTUs. Correlations were calculated using the Sparse Correlations for Compositional data algorithm (SparCC), and p-values calculated using 1000 bootstraps (Friedman and Alm, 2012). Networks were produced by retaining edges with a correlation ≥ ±0.8 and p ≤ 0.01 and were visualized using igraph (Csardi and Nepusz, 2006) and ggplot2. Networks were sorted into clusters using the fast-greedy algorithm (Clauset et al., 2004).
Field Assessment of Pathogen Infection of Stems and Pods
Diseases on stems and pods caused by different fungal pathogens were assessed before harvest on 18/06/2014 by sampling six plants per plot. Phoma stem canker (caused by Leptospaeria maculans and L. biglobosa), was assessed by cutting the stem just above the soil and scoring the severity of symptoms at the stem base (crown) on a 0–7 scale (1 < 5% stem area diseased, 2 < 25%, 3 < 50%, 4 < 75%, 5 < 100%, 6 = 100% but upper stem green/firm, 7 = 100% but plant dead/dried/broken). Upper stem lesions, which are generally caused by Leptosphaeria spp., were also scored using the same scale. Light leaf spot, caused by Pyrenopeziza brassicae, was assessed on stems and the pod canopy by estimating the percent area of stem or pods affected. Dark pod spot (generally caused by Alternaria brassicae and/or A. brassicola) was assessed by recording the appearance or absence of the disease. The effect of nitrogen and genotype on phoma stem canker and light leaf spot was determined using Analysis of Variance.
Results
Microbial Alpha and Beta Diversity
For bacteria, fungi and protists, Fisher's alpha diversity was significantly (p = 1.9−11, p = 2.5−11, p = 2.7−11, respectively) higher in soil relative to the rhizosphere, while for nematodes there was no significant difference (p = 0.53) (Supplementary Figure 1). Nitrogen treatment had no significant effect on Fisher's alpha diversity in soil or rhizosphere for any microbial group, and had no significant effect on microbial communities at the phyla level (bacteria and fungi) or class level (protists and nematodes) (Supplementary Figure 2). OSR genotype had no significant effect on Fisher's alpha diversity in soil or rhizosphere for any microbial group (data not shown).
PERMANOVA (Table 2) and NMDS analysis (Figure 2) showed that bacterial, fungal, protist and nematode beta diversity were significantly different (p < 0.001) between the roots and rhizosphere. Overall, nitrogen significantly affected bacterial (p = 0.032) fungal (p = 0.029) and protist (p = 0.045) beta diversity, but had no significant effect on nematode communities (p = 0.34). However, while compartment (rhizosphere vs. soil) accounted for between 23 and 42% of community variation, nitrogen treatment explained <3% of variation for fungi, bacteria and protists. OSR genotype significantly (p = 0.035) affected fungal beta diversity, accounting for 14.6% of community variation, but had no significant effect on the other microbial groups (Supplementary Figure 3, Table 2). For fungi only there was a significant (p = 0.037) interaction between nitrogen treatment and compartment, suggesting that for fungi, but not bacteria and protists, nitrogen had different effects on beta diversity in the soil and rhizosphere. There was no significant relationship between genetic similarity of OSR genotypes and the similarity of their microbial community (fungi p = 0.21, bacteria p = 0.24, protists p = 0.2, and nematodes p = 0.39).
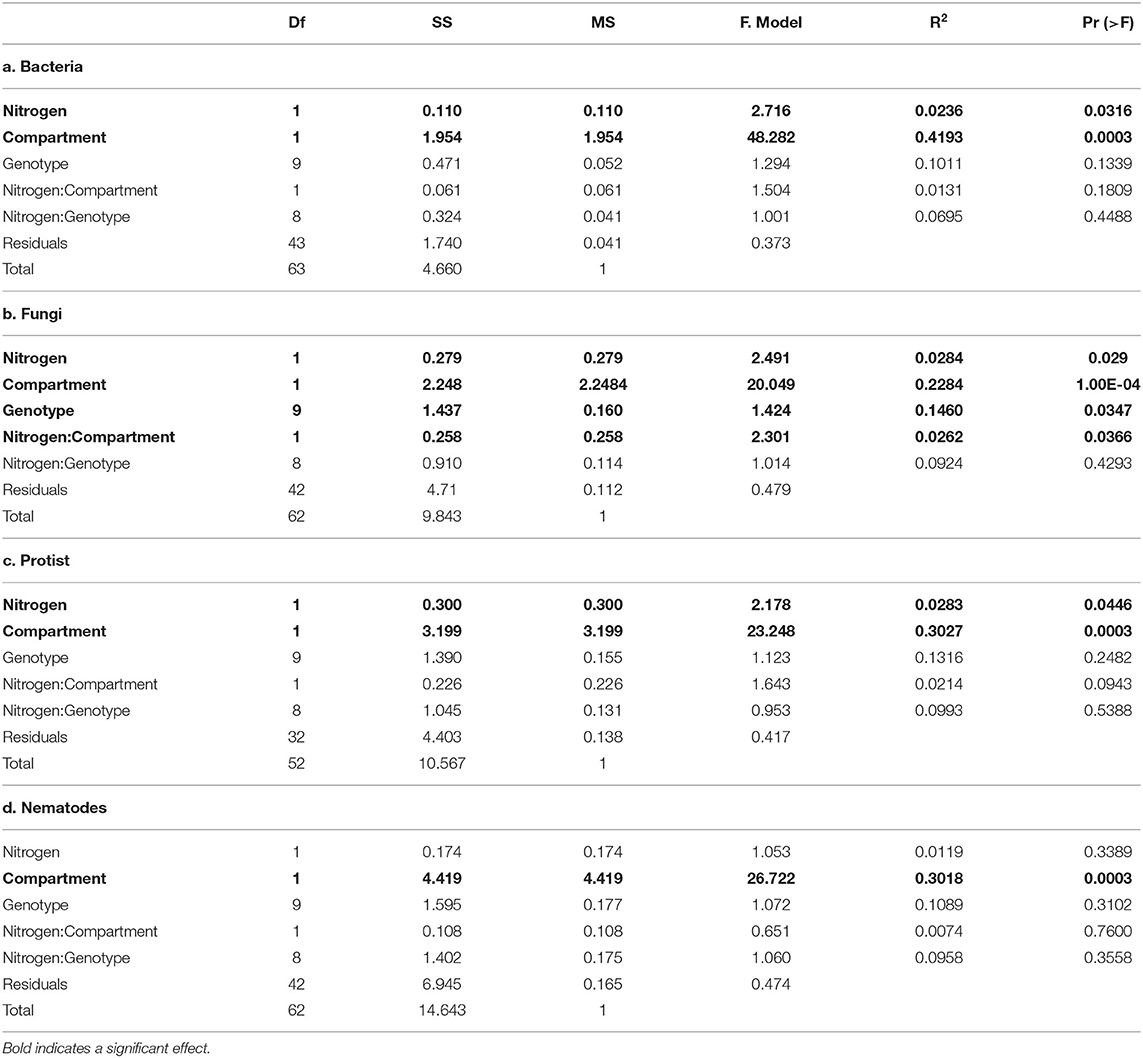
Table 2. Permutational multivariate analysis of variance (PERMANOVA) of the effect of nitrogen treatment, oilseed rape genotype and compartment (soil vs. rhizosphere), and their interactions, on microbial community composition.
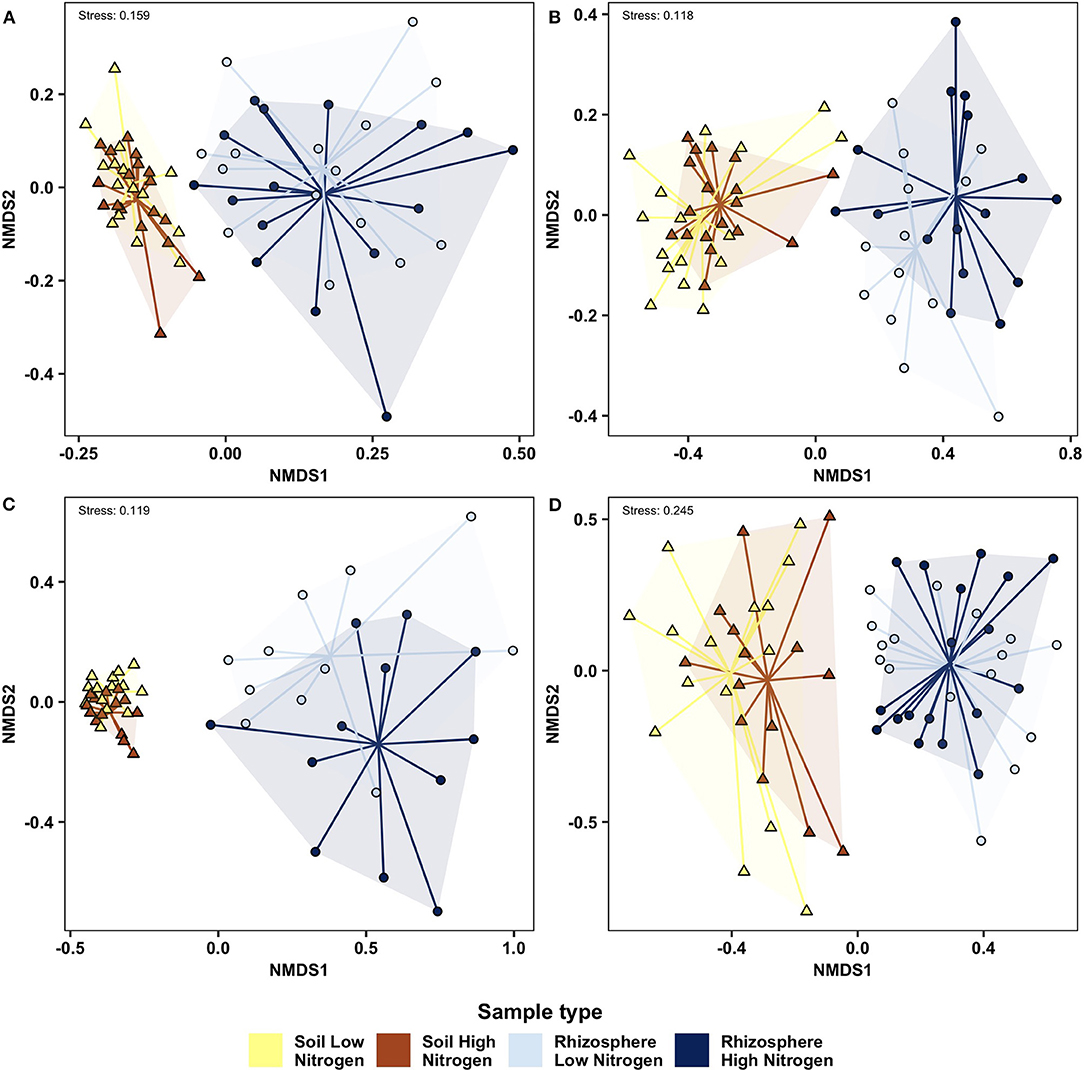
Figure 2. Non-metric multidimensional scaling analysis of soil and rhizosphere microbial communities determined using Bray Curtis similarity analysis of high and low nitrogen treatments, with data for each treatment reflecting combined data from 10 oilseed rape genotypes. (A) Bacteria; (B) Fungi; (C) Protist; (D) Nematode. Samples from each compartment/ nitrogen treatment combination are connected to a group centroid inside colored segments. Δ, soil; o, rhizosphere.
SIMPER analysis revealed that the key OTUs that contributed most to the difference in fungal beta diversity between the rhizosphere of high and low nitrogen treatments included the pathogens Leptosphaeria maculans F2 (12.3%), Mycosphaerella sp. F3 (11.9%), Pyrenopeziza brassicae F6 (5.5%), Olpidium brassicae F40 (4.1%), and L. biglobosa F35 (3%). These fungi all showed higher relative abundance in the high nitrogen compared to the low nitrogen treatment (Table 3). Together, these OTUs accounted for 48.1% of fungal relative abundance in the high nitrogen treatment, compared to 33.8% in the low nitrogen treatment. Mycosphaerella sp. F3 showed the greatest change in relative abundance, accounting for 18.8% of fungal relative abundance in the high nitrogen treatment, compared to 11.9% in the low nitrogen treatment. Similarly, the endophyte Tetracladium sp. F22 (4.6%) increased in abundance in the high nitrogen relative to low nitrogen treatment, with Apodus sp. F32 (3%) showing the reverse trend. Similarly, Mycosphaerella sp. F3 (8.2%), L. maculans F2 (6.6%), and P. brassicae F6 (3.1%), together with Gibellulopsis nigrescens F4 (3.1%), were key contributors to community dissimilarity between high and low nitrogen treatments in the soil.
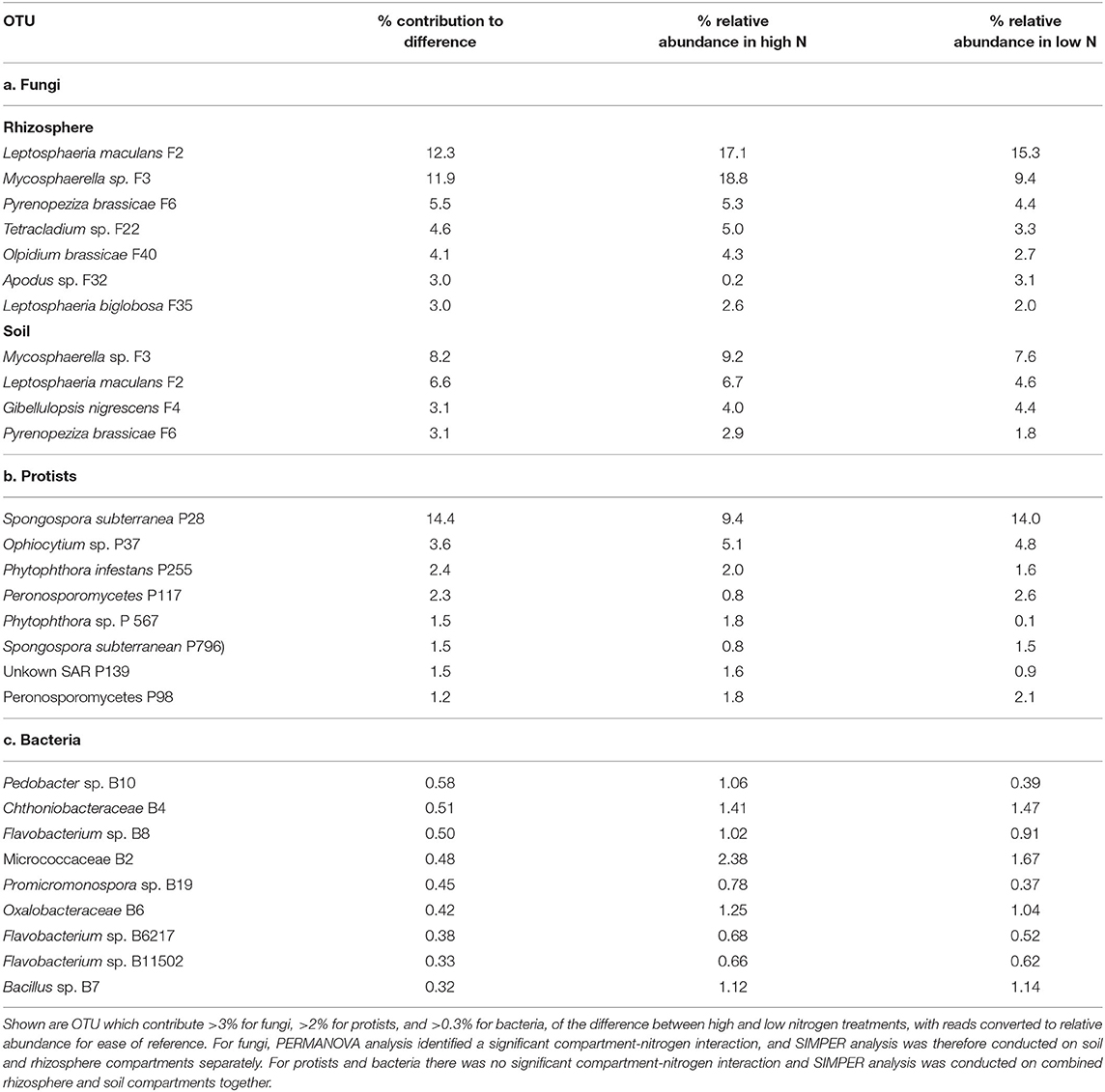
Table 3. Analysis of similarity percentage (SIMPER) of differences in oilseed rape rhizosphere and soil fungal, protest, and bacterial OTU between high and low nitrogen treatments (with all 10 oilseed rape genotypes combined).
For the protists, differences in rhizosphere beta diversity between the high and low nitrogen treatments was associated particularly with a change in relative abundance of Spongospora subterranea P28 (14.4%), which comprised 14% of protist relative abundance under low nitrogen, but only 9.4% under high nitrogen. Additionally, Phytophthora sp. OTUs P255 and P567 contributed 2 and 1.8% of dissimilarity between nitrogen treatments, with both showing higher relative abundance in the high nitrogen treatment relative to the low nitrogen treatment.
For bacteria, OTU which contributed >0.3% of dissimilarity between nitrogen treatments included Pedobacter sp. (B10), Flavobacterium sp. (B8), Micrococcaceae (B2), Promicromonospora sp. (B19), and Oxalobacteriaceae (B6) which all had higher relative abundance in the low relative to high nitrogen treatment, and Chthoniobacteraceae (B4) which showed the reverse trend.
Differences in fungal beta diversity between crop genotypes was associated particularly with distribution of the pathogen Leptosphaeria maculans, which contributed 14.49% to the difference between genotypes (Table 4). This fungus accounted for over 22% of relative abundance in Couve, Yudal, Tapidor and Ningou, but only 2% of relative abundance in Canard and York. Similarly, Mycosphaerella sp. contributed 13.97% to the difference between genotypes and comprised over 23% relative abundance in Victor and Ningyou, but <10% relative abundance in Tapidor. Furthermore, the pathogens Pyrenopeziza brassicae, Olpidium brassicae, and Leptosphaeria biglobosa contributed 6.10, 4.36, and 3.51% to the difference between genotypes, associated with marked differences in relative abundance. P. brassicae was highly abundant (15.3%) in Darmoor but comprised <1% relative abundance in Rocket, Temple, and York, while O. brassicae comprised 10.5% relative abundance in Rocket, and just 1.4% relative abundance in Temple. L. biglobosa varied between 8.71% in Tapidor and 0.33% in Victor.
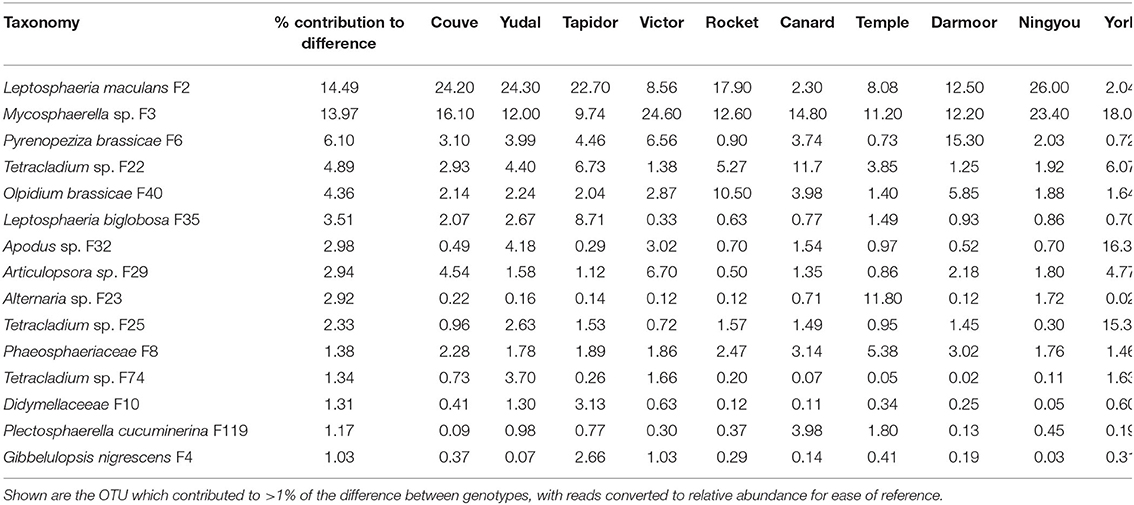
Table 4. Analysis of similarity percentage (SIMPER) of differences in oilseed rape rhizosphere fungal OTU between oilseed rape genotypes (high and low nitrogen treatments combined).
Interestingly, three Tetracladium sp. OTU, when combined contributed over 8.5% to the difference between genotypes. These OTUs showed considerable differences in relative abundance between genotypes; F22 was highly abundant (11.7%) in Canard, F25 in York (15.3%) and F74 in Yudal (3.7%), with each being present in very low abundance in some specific genotypes. A number of other OTUs contributed to over 2% of genotype dissimilarity, and showed high relative abundance in specific genotypes, including Apodus sp., which was abundant in York (16.34%), Articulospora sp., which was abundant in Victor (6.70%), and Alternaria sp., which was abundant in Temple (11.80%).
OTU Indicators of Nitrogen Treatment and Crop Genotype
Indicator analysis was used to identify OTUs which were significantly associated with nitrogen treatment in the soil and rhizosphere. For all taxonomic groups, there was limited overlap between specific OTU indicators of nitrogen treatment in the rhizosphere and soil (Supplementary Figure 4, Supplementary File 1). Most microbial phyla possessed indicators for both high and low nitrogen treatments (Supplementary Figure 5). However, in the rhizosphere, indicators of low nitrogen were particularly associated with the bacterial phyla Amatimonadetes and Acidobacteria, the protist group Rhizaria, and the nematode classes Enoplea and Chromaonea. In contrast, rhizosphere indicators of high nitrogen were associated with the bacterial phyla TM7, Proteobacteria, Firmicutes, Bacteroidetes and Actinobacteria, the chytridiomycete and ascomycete fungi, and the protist Stramenopiles.
In the soil, groups possessing indicators of high nitrogen largely matched those in the rhizosphere, but a broader range of bacterial phyla were enriched as indicators of low nitrogen, including Verrucomicrobia, Gemmatimonadetes, Cyanobacteria, Chloroflexi, Chlorobi, BRC1, and Aramatimonadetes.
Generally, most indicators for B. napus genotype were common across a number of genotypes (Supplementary Figure 5, Supplementary File 1). Canard and York had the most distinctive indicators: Canard had 2 fungal indicators which were unique to it, and Tapidor a single unique fungal indicator. A chytrid-like OTU, Sanchytrium tribonematis had over 75% of its total relative abundance in Ningyou 7, while there were 11 fungal indicators which had over 50% of their total relative abundance in York, including two Tetracladium sp. OTU.
Generally, there was limited overlap between taxa the SIMPER analysis identified as contributing to the differences in communities between nitrogen treatment or genotype, and indicator species (Supplementary File 1). However, for fungal indicators, there was some overlap with results of the SIMPER analysis, with L. maculans and L. biglobosa indicators of high nitrogen rhizosphere soil. However, most OTU identified by the indicator analysis had low relative abundance.
Effects of Nitrogen Treatment on Microbial Networks
Network density was increased by nitrogen application in both the rhizosphere and soil, as shown in network co-occurrence statistics (Table 5) and visually in the microbial correlation networks (Figure 3). High nitrogen was associated with a reduction in the number of nodes, from 895 and 726 to 639 and 592 in the rhizosphere and soil, respectively. However, there was no substantive difference in the relative proportion of bacterial, protist, fungal or bacterial OTUs within networks from the high and low nitrogen treatments. Notably, the proportion of fungal OTU within networks was markedly lower in the rhizosphere (7.6–7.7%) relative to the soil (14.2 and 13.5%). The proportion of positive network connections in the soil was similar in the high and low nitrogen treatments (26.8 and 27.9%, respectively), but in the rhizosphere, there was over a 5% difference in the proportion of positive network connections between the high (27.7%) and low (22.4%) nitrogen treatments.
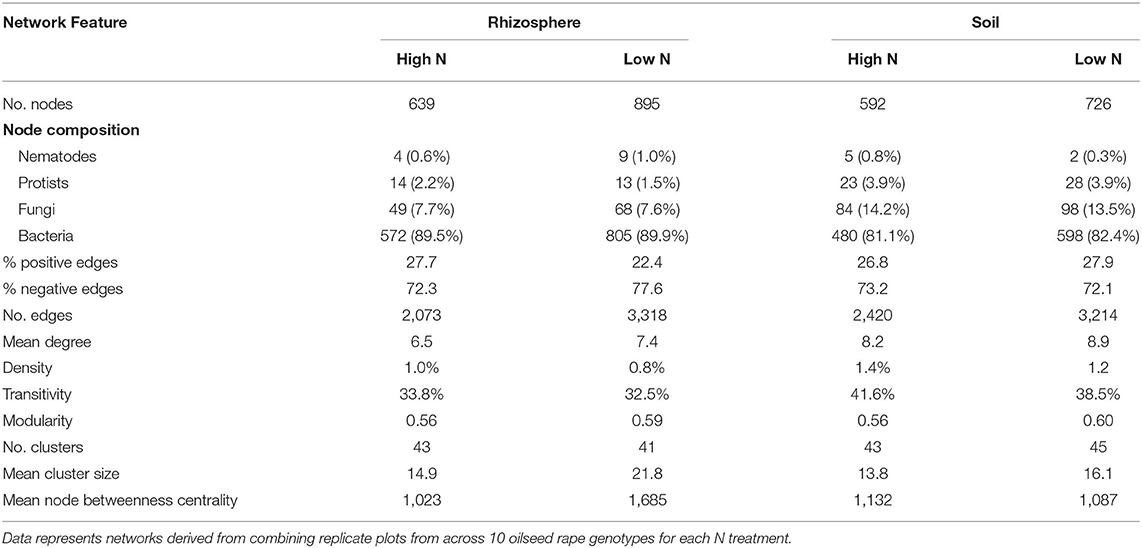
Table 5. Network co-occurrence statistics in the soil and rhizosphere of oilseed rape crops under high and low nitrogen fertilization treatments.
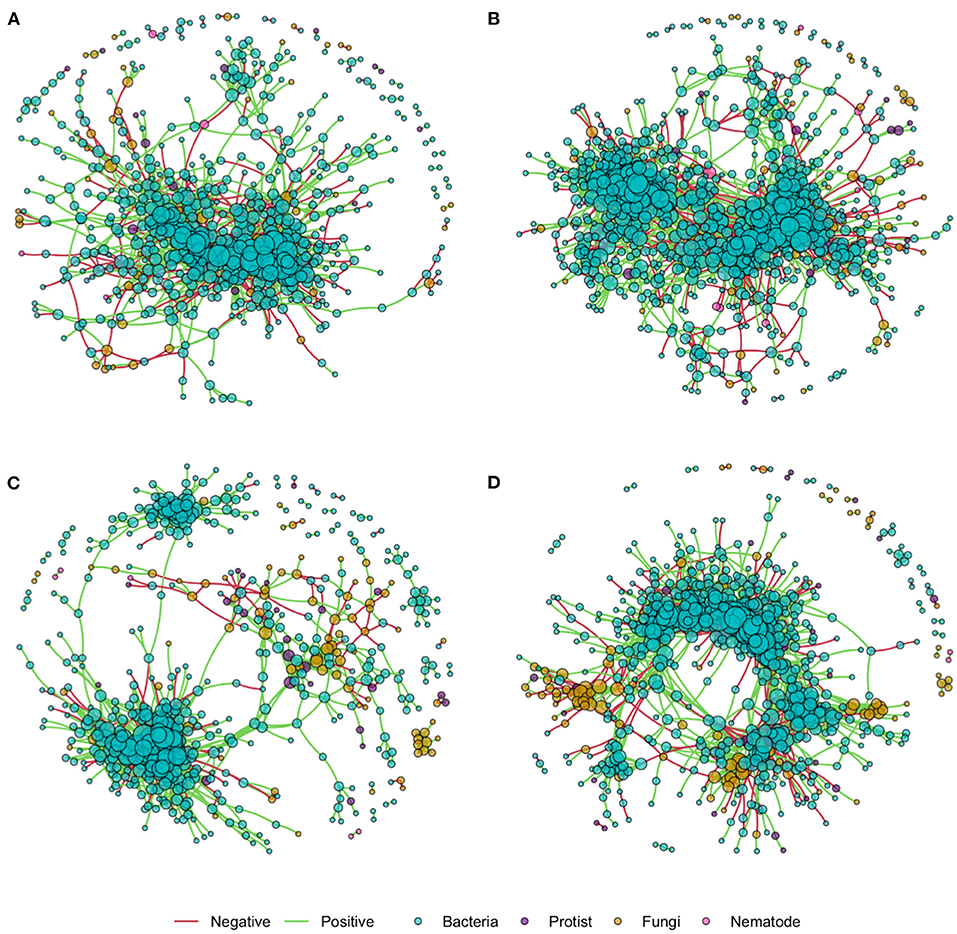
Figure 3. Inter-kingdom microbial correlation networks in the rhizosphere and soil of oilseed rape in high and low nitrogen treatments, using data from 10 oilseed rape genotypes for each nitrogen treatment. Edges indicate correlation of >0.8 or <-0.8. (A) Rhizosphere high N; (B) Rhizosphere low N; (C) Soil high N; (D) Soil low N.
The number of edges was reduced by nitrogen application, from 3,318 and 3,214, to 2,073 and 2,420 in the rhizosphere and soil, respectively. Similarly, nitrogen application reduced the mean degree, but increased density and mean cluster size. Mean node betweenness centrality was markedly affected by nitrogen in the rhizosphere, where it was reduced by 40% in the high nitrogen relative to the low nitrogen treatment. Modularity, the number of clusters and transitivity showed limited responses to nitrogen treatment in the soil or rhizosphere.
Visual Assessment of Pathogen Infection of Stems and Pods in the Field
Phoma stem canker and light leaf spot were detected on all oilseed rape genotypes (Supplementary Table 2). Alternaria spp. (A. brassicae and/or A. brassicola) was also found to be present on most plants, although infection levels were not scored. There was no significant effect of nitrogen treatment on infection severity of phoma stem canker (p = 0.71) or upper stem lesions (p = 0.98). Similarly nitrogen treatment had no effect on % stem (p = 0.12) or pod (p = 0.06) covered by light leaf spot. Genotype had a significant effect on both phoma stem canker (p = 2−8) and upper lesion severity (p = 3−4). Both of these analyses showed high levels of infection in Victor, and low infection levels in Temple and Ningyou 7, but for the other genotypes there was no relationship between severity of stem canker and upper stem lesions. Canard and Couve Nabica showed high stem canker infection rates, but low rates of upper stem lesions, while Darmoor showed the reverse trend. Genotype had no significant effect on light leaf spot infection of the stem (p = 0.07) or pods (p = 0.12).
Discussion
We show that while bacteria, fungi, nematodes, and protists showed distinct communities in the rhizosphere of OSR, relative to the bulk soil, there were contrasting responses among these microbial groups to both crop genotype and nitrogen fertilization. Nematode communities were unaffected by either nitrogen application or crop genotype. Fungal community composition was affected by both factors, while protists and bacteria were influenced by fertilization but not crop genotype. Significantly, for fungi, the effect of nitrogen application on community composition was associated with an increase in relative abundance of a variety of plant pathogens. Impacts of crop genotype on fungal community composition were mediated through specific fungal taxa, particularly pathogens, which were differentially abundant across genotypes. However, there was no relationship between relatedness of B. napus genotypes and the similarity of their microbial communities.
Availability of nitrogen has the potential to directly influence microbial communities by affecting nutrient stoichiometry, which can change the nature of nutrient related resource limitations, and thereby affect microbial growth, and the rates and characteristics of carbon and nutrient cycling processes (Aanderud et al., 2018). In the rhizosphere such effects can also occur indirectly, since changes in plant nitrogen status can cause quantitative and qualitative changes in the composition of plant root exudates, thereby affecting microbial community composition (Zhu et al., 2016).
Nitrogen fertilizer application has previously been found to affect soil and rhizosphere bacterial communities in a number of crops, including soybean (Wang et al., 2020), rice (Chen et al., 2019), OSR (Monreal et al., 2018), and maize (Zhu et al., 2016). However, the effects of nitrogen application on bacterial communities may be small relative to other factors (Wang et al., 2016) and some studies have found no influence of nitrogen application on rhizosphere bacteria (Revillini et al., 2019). Evidence suggests that bacteria involved in nitrogen cycle transformations are most responsive to nitrogen application in both the rhizosphere and bulk soil (Kastl et al., 2014; Zhu et al., 2016), and indeed Nitrospirae were among the OTU indicators of high nitrogen in our study.
Much less is known about the influence of nitrogen on microbial eukaryotes in the soil or rhizosphere. There is some evidence that nitrogen fertilizer application can reduce diversity of bulk soil nematode communities, potentially by affecting pH (Liang et al., 2020). Furthermore, Revillini et al. (2019) found that abundance of arbuscular mycorrhizal fungi was reduced by nitrogen application, and similarly a strong effect of nitrogen application on rhizosphere fungi has been seen in sugarcane (Paungfoo-Lonhienne et al., 2015). Significantly, our data suggests differential impacts of nitrogen fertilization on fungal communities, but not protists, between the rhizosphere and soil.
Overall these findings suggest contrasting responses among different groups of soil biota to nitrogen fertilization, and the extent to which the plant influences these effects. Changes in nitrogen availability from fertilization were not of sufficient magnitude to cause stoichiometric shifts suitable to alter growth of nematode communities, but did impact protist, bacterial and fungal communities, with effects modulated by the plant for fungi but not protists or bacteria. Clearly the extent to which such effects will occur for any microbial group will depend on local soil stoichiometry and the characteristics of plant exudates, but our data does suggest differential susceptibility among soil biota to these thresholds.
The nematode and protist communities were profiled by extracting sequenes amplified using general microbial eukaryote primers. While this represents a low cost option relative to use of specific primers for these groups, such as those used by Hilton et al. (2018) for nematodes, and by Bass et al. (2018) for plant associated plasmodiophorid protists, it is possible that some loss of diversity within these groups occurred, resulting in dampening of the responses of these groups to both nitrogen and genotype.
Notably, nitrogen fertilization was associated with increases in relative abundance of a number of pathogens in the rhizosphere of OSR. This included Leptosphaeria maculans and L. biglobosa, which cause blackleg diseases which impact OSR yields globally (Fitt et al., 2006), Pyrenopeziza brassicae, responsible for light leaf spot, the predominant OSR disease in the UK (Dewage et al., 2018), Olpidium brassicae, which is associated with OSR yield decline (Hilton et al., 2013, 2021), and Mycosphaerella sp. which are common plant pathogens and includes several species which infect oilseed rape, such as M. brassicola (ringspot disease) and M. capsellae (white leaf spot disease). Nitrogen fertilization may play a pivotal role in plant defense (Mur et al., 2016). While nitrogen availability generally promotes plant defence metabolites, it may cause changes in host physiology, such as promotion of sugar content, which can increase resource availability to pathogens. Furthermore, pathogens may be nitrogen limited when they grow toward and within plant tissues, and increased nitrogen availability may thus increase the rate of infection.
With the exception of O. brassicae, the pathogens we detected cause foliar diseases, and the importance of root entry in driving disease development is unclear, since the presence of pathogens in the rhizosphere can reflect growth from the shoot (Sprague et al., 2007). However, for Mycosphaerella sp., L. maculans, and P. brassicae, the high nitrogen treatment was also associated with increased relative abundance in the bulk soil, suggesting that high nitrogen could have promoted survival or growth of spores, or mycelium residing on the debris of previous crops.
Overall, pathogens were dominant components of the OSR root fungal microbiome, and levels of infection appear to be linked to nutrient stoichiometry, which influenced pathogen communities in both the soil and rhizosphere. Our results suggest that nitrogen application could be an important contributor to determining whether microbiomes have positive or negative impacts on crop health.
Relative to the bulk soil, the rhizosphere network was characterized by a variety of features which indicate lower connectivity, including lower mean degree, density and transitivity, while number of nodes, showed the reverse trend. Nitrogen application reduced number of nodes, edges, mean degree and cluster size, suggesting reduced connectivity, and furthermore in the rhizosphere only, nitrogen application reduced mean node betweenness centrality, and increased the number of positive connections. The interpretation and significance of network features and their thresholds in microbiomes is currently unclear, although it has been proposed that features which confer stability and resilience to perturbation in microbiomes may differ from those in ecological networks, because of the fundamental importance of cooperative interactions in the functioning of microbiomes (Coyte et al., 2015). However, high connectivity, centrality and positive interactions have all been proposed to reduce microbial network stability (Coyte et al., 2015; de Vries et al., 2018), indicating that communities of the rhizosphere have greater inherent stability than those in the bulk soil, but that nitrogen application reduces community stability within the rhizosphere.
Host genotype exerted a significant effect on fungal community assembly, while no such effect was seen for bacteria, nematodes or protists. In common with other crop species, studies of the role of host genetics in shaping the OSR microbiome have largely focused on bacterial communities (Taye et al., 2020). However, evidence suggests that plant rhizosphere bacterial communities may show low responsiveness to host genotype under field conditions, with stronger responses noted in the phyllosphere (Edwards et al., 2015; Wagner et al., 2016). However, bacterial responses to genotype may be determined by plant development stage (Taye et al., 2020).
Significantly there was no relationship between genotype relatedness and the similarity of microbiomes. Fitzpatrick et al. (2018) showed that similarity of the root, but not rhizosphere, bacterial microbiome in 30 plant species grown under controlled conditions was associated with plant genetic similarity. Furthermore, using a field approach, Taye et al. (2020) demonstrated a correlation between the similarity of bacterial communities in the OSR rhizosphere and plant genetic distance.
Differences in responsiveness of microbiota to host genotype likely reflects the nutritional mode of the interaction, and the degree to which this is affected by plant traits under the influence of genotype, such as the presence of defence and nutritional metabolites, such as glucosinolates and erucic acid. In our study, fungal communities were dominated by plant pathogens. Notably, differences in fungal community composition between genotypes was associated with differences in relative abundance of several pathogens, including Leptosphaeria maculans, Alternaria sp., Pyrenopeziza brassicae, Olpidium brassicae, Mycosphaerella sp., and Leptosphaeria biglobosa between genotypes. This could indicate differences in genetic resistance to the pathogens, or reflect assembly of microbiomes which inhibit pathogen assembly on the root (Glaeser et al., 2019).
Visual analysis of all oilseed rape genotypes confirmed that they were infected by Leptosphaeria sp., P. brassicae and Alternaria sp., as indicated by the rhizosphere community profiling. However, there was no relationship between the severity of infection of these pathogens in aboveground plant tissues and the relative abundance of these pathogens within the rhizosphere microbiome, and similarly, while sequencing revealed a potential relationship between relative abundance of these fungal pathogens and nitrogen application, no such relationship was seen in the visual disease analysis. Since analysis of disease severity on stems and pods was not concomitant with the analysis of rhizosphere microbial communities, comparison should be treated with caution. However, our findings clearly demonstrate that rhizosphere microbiome composition is a direct indicator of plant health, and identified the presence of pathogens that were not detected by visual analysis.
Interestingly, Darmor and Tapidor genotypes have been bred for genetic resistance to L. maculans, while Ningyou 7 and Yudal are noted as susceptible to this pathogen (AHDB, 2015; Larkin et al., 2015; Raman et al., 2018). Microbiome analysis confirmed high relative abundance of L. maculans sequences in Ningyou 7 and Yudal relative to Darmor, but not Tapidor. Furthermore, aboveground disease analysis did not identify differences in infection severity on stems or pods between these lines. Similarly, there was no evidence for greater susceptibility of Tapidor to P. brassicae than the other lines, as suggested by Larkin et al. (2015). These specific pathogen resistance traits cannot therefore be used to predict infection by these pathogens either in the roots or aboveground, and in particular questions the effectiveness of L. maculans resistance traits under field conditions.
Fungal root endophytes have frequently been proposed as potential plant pathogen biocontrol agents (Whipps, 2001). Interestingly the genotype York showed the lowest amount of L. maculans, P. brassicae, O. brassicae, and L. biglobosa, with a combined total of 5.1% relative abundance, compared to 37.9% in Tapidor. In contrast to the other genotypes, in which non-pathogen fungal OTUs were each generally present in low abundance, York supported extremely high relative abundance of Apodus sp., and Tetracladium sp. F25, which together comprised almost 32% of fungus relative abundance. Tetracladium spp. have traditionally been considered as aquatic hyphomycetes, but evidence is emerging, supported here, that they are widely distributed root endophytes (Selosse et al., 2008) which may be indicators of a beneficial microbiome (Hilton et al., 2021). While there is no understanding of the interactions between these fungi with their host or plant pathogens, some related Heliotales may act as root symbionts which promote plant growth and nutrient supply (Almario et al., 2017).
A variety of approaches are used to profile responses of the microbiome to variables such as environment and host. Typically, these include analysis of alpha and beta diversity as used here. Although these methods are useful to identify broad relationships, they have the potential to overlook behaviours of individual taxa, and also the responses of microbial interaction pathways (Tabrett and Horton, 2020). Indicator species analysis, which accounts for both abundance and frequency of taxa detection, and is calculated independently for each taxon, is becoming widely used to investigate microbiome composition. We found that indicator species analysis identified complex OTU level responses, particularly for nitrogen treatment, but there was limited overlap between indicator OTUs and OTUs identified by SIMPER as contributing to community level changes. Nonetheless indicator analysis and particularly network analysis may be useful in providing detail about fine scale changes in community composition which could have biological significance.
We conclude that factors which shape composition of bacterial communities in the rhizosphere cannot be extrapolated to microbial eukaryotes, with bacteria, fungi, protists, and nematodes all showing variable responses to the factors we studied. We identified fungal communities as the most responsive to host genotype and in contrast to the other groups, found that impacts of nitrogen on the microbiome were modulated by the plant. Both nitrogen and genotype contributed to fungal assembly patterns which could influence host health. While controlled environment experiments have been important for identifying factors which have potential to contribute to microbiome assembly, field-based approaches are required to unravel the complex interactions which shape microbiomes in the real world.
Data Availability Statement
The datasets presented in this study can be found in online repositories. The names of the repository/repositories and accession number(s) can be found here: https://www.ncbi.nlm.nih.gov/genbank/, MW051022-MW051025 https://www.ncbi.nlm.nih.gov/genbank/, MW051900-MW051914 https://www.ncbi.nlm.nih.gov/, BioProject ID PRJNA666392.
Author Contributions
CH: rhizosphere sampling. CH and SH: microbial sequencing. CH, EP, and SH: bioinformatic analysis. Y-JH and SP: crop disease assessment. GT: crop genotype SNP analysis. CH and GB: writing the manuscript. GB: project conception and obtaining project funding. All authors edited the manuscript.
Conflict of Interest
The authors declare that the research was conducted in the absence of any commercial or financial relationships that could be construed as a potential conflict of interest.
Acknowledgments
We thank the Biotechnology and Biological Sciences Research Council grants BB/J019690/1 and BB/L025892/1 and the Defra OREGIN project (IF0144) for funding.
Supplementary Material
The Supplementary Material for this article can be found online at: https://www.frontiersin.org/articles/10.3389/fsufs.2021.613269/full#supplementary-material
References
Aanderud, Z. T., Saurey, S., Ball, B. A., Wall, D. H., Barrett, J. E., Muscarella, M. E., et al. (2018). Stoichiometric shifts in soil C:N:P promote bacterial taxa dominance, maintain biodiversity, and deconstruct community assemblages. Front. Microbiol. 9:1401. doi: 10.3389/fmicb.2018.01401
AHDB (2015). Final Report 606 Understanding Resistance to Decrease Risk of Severe Phoma Stem Canker in Oilseed Rape. Agriculture and Horticulture Development Board.
Almario, J., Jeena, G., Wunder, J., Langen, G., Zuccaro, A., Coupland, G., et al. (2017). Root-associated fungal microbiota of nonmycorrhizal Arabis alpina and its contribution to plant phosphorus nutrition. Proc. Natl. Acad. Sci. U.S.A. 114, E9403–E9412. doi: 10.1073/pnas.1710455114
Amaral-Zettler, L. A., McCliment, E. A., Ducklow, H. W., and Huse, S. M. (2009). A method for studying protistan diversity using massively parallel sequencing of V9 hypervariable regions of small-subunit ribosomal RNA Genes. PLoS ONE 4:e6372. doi: 10.1371/annotation/50c43133-0df5-4b8b-8975-8cc37d4f2f26
Bass, D., van der Gast, C. J., Thomson, S., Neuhauser, S., Hilton, S., and Bending, G. D. (2018) Plant rhizosphere selection of plasmodiophorid lineages from bulk soil: the importance “hidden” diversity. Front. Microbiol. 9:168. doi: 10.3389/fmicb.2018.00168
Bolger, A. M., Lohse, M., and Usadel, B. (2014). Trimmomatic: a flexible trimmer for Illumina sequence data. Bioinformatics 30, 2114–2120. doi: 10.1093/bioinformatics/btu170
Bulgarelli, D., Garrido-Oter, R., Münch, P., Weiman, A., Dröge, J., Pan, Y., et al. (2015). Structure and function of the bacterial root microbiota in wild and domesticated barley. Cell Host Microbe 17, 392–403. doi: 10.1016/j.chom.2015.01.011
Caporaso, J. G., Kuczynski, J., Stombaugh, J., Bittinger, K., Bushman, F. D., Costello, E. K., et al. (2010). QIIME allows analysis of high-throughput community sequencing data. Nat. Methods 7, 335–336. doi: 10.1038/nmeth.f.303
Caporaso, J. G., Lauber, C. L., Walters, W. A., Berg-Lyons, D., Huntley, J., Fierer, N., et al. (2012). Ultra-high-throughput microbial community analysis on the Illumina HiSeq and MiSeq platforms. ISME J. 6, 1621–1624. doi: 10.1038/ismej.2012.8
Chen, J., Arafat, Y., Ud Din, I., Yang, B., Zhou, L., Wang, J., et al. (2019). Nitrogen fertilizer amendment alter the bacterial community structure in the rhizosphere of rice (Oryza sativa L.) and improve crop yield. Front. Microbiol. 10:2623. doi: 10.3389/fmicb.2019.02623
Clauset, A., Newman, M. E. J., and Moore, C. (2004). Finding community structure in very large networks. Phys. Rev. E 2004:70. doi: 10.1103/PhysRevE.70.066111
Coyte, K. Z., Schluter, J., and Foster, K. R. (2015). The ecology of the microbiome: networks, competition, and stability. Science 350, 663–666. doi: 10.1126/science.aad2602
Csardi, G., and Nepusz, T. (2006). The Igraph Software Package for Complex Network Research, 1695. Available online at: http://igraph.org InterJournal Complex Systems
de Vries, F. T., Griffiths, R. I., Bailey, M., Craig, H., Girlanda, M., Gweon, H. S., et al. (2018). Soil bacterial networks are less stable under drought than fungal networks. Nat. Commun. 9:3033. doi: 10.1038/s,41467-018-05516-7
DEFRA: Agriculture in the United Kingdom. (2019). National Statistics, Department for Environment, Food & Rural Affairs.
Dewage, C. S. K., Klöppel, C. A., Stotz, H. U., and Fitt, B. D. L. (2018). Host–pathogen interactions in relation to management of light leaf spot disease (caused by Pyrenopeziza brassicae) on Brassica species. Crop and Pasture Science 69, 9–19. doi: 10.1071/CP16445
Dufrêne, M., and Legendre, P. (1997). Species assemblages and indicator species: the need for a flexible asymmetrical approach. Ecol. Monogr. 67, 345–366. doi: 10.1890/0012-9615(1997)0670345:SAAIST2.0.CO;2
Edgar, R. C. (2010). Search and clustering orders of magnitude faster than BLAST. Bioinformatics 26, 2460–2461. doi: 10.1093/bioinformatics/btq461
Edgar, R. C. (2013). UPARSE: highly accurate OTU sequences from microbial amplicon reads. Nat. Methods 10:996. doi: 10.1038/nmeth.2604
Edwards, J., Johnson, C., Santos-Medellín, C., Lurie, E., Podishetty, N. K., Bhatnagar, S., et al. (2015). Structure, variation, and assembly of the root-associated microbiomes of rice. Proc. Natl. Acad. Sci. U.S.A. 112, E911–E920. doi: 10.1073/pnas.1414592112
Fitt, B. D. L., Brun, H., Barbetti, M. J., and Rimmer, S. R. (2006). World-wide importance of phoma stem canker (Leptosphaeria maculans and L. biglobosa) on Oilseed Rape (Brassica napus). Europ. J. Plant Pathol. 114, 3–15. doi: 10.1007/s10658-005-2233-5
Fitzpatrick, C. R., Copeland, J., Wang, P. W., Guttman, D. S., Kotanen, P. M., and Johnson, M. T. J. (2018). Assembly and ecological function of the root microbiome across angiosperm plant species. Proc. Natl. Acad. Sci. U.S.A. 115, E1157–E1165. doi: 10.1073/pnas.1717617115
Friedman, J., and Alm, E. J. (2012). Inferring correlation networks from genomic survey data. PLoS Comput. Biol. 8:e1002687. doi: 10.1371/journal.pcbi.1002687
Gao, Z., Karlsson, I., Geisen, S., Kowalchuk, G., and Jousset, A. (2019). Protists: puppet masters of the rhizosphere microbiome. Trends Plant Sci. 24, 165–176. doi: 10.1016/j.tplants.2018.10.011
Glaeser, S. P., Gabur, I., Haghighi, H., Bartz, J., Kämpfer, P., Snowdon, R., et al. (2019). Endophytic bacterial communities of oilseed rape associate with genotype-specific resistance against Verticillium longisporum. FEMS Microbiol. Ecol. 96:fiz188. doi: 10.1093/femsec/fiz188
Gosling, P., Hodge, A., Goodlass, G., and Bending, G. D. (2006). Arbuscular mycorrhizal fungi and organic farming. Agricult. Ecosyst. Environ. 113, 17–35. doi: 10.1016/j.agee.2005.09.009
Guillou, L., Bachar, D., Audic, S., Bass, D., Berney, C., Bittner, L., et al. (2013). The Protist Ribosomal Reference database (PR2): a catalog of unicellular eukaryote Small Sub-Unit rRNA sequences with curated taxonomy. Nucleic Acids Res. 41, D597–D604. doi: 10.1093/nar/gks1160
Hamilton, N. E., and Ferry, M. (2018). ggtern: ternary diagrams using ggplot2. J. Stat. Softw. 87, 1–17. doi: 10.18637/jss.v087.c03
Hammer, Ø., Harper, D. A. T., and Ryan, P. D. (2001). PAST: Paleontological statistics software package for education and data analysis. Palaeontol. Electro. 4:9.
Hilton, S., Bennett, A. J., Chandler, D., Mills, P., and Bending, G. D. (2018). Preceding crop and seasonal effects influence fungal, bacterial and nematode diversity in wheat and oilseed rape rhizosphere and soil. Appl. Soil Ecol. 126, 34–46. doi: 10.1016/j.apsoil.2018.02.007
Hilton, S., Bennett, A. J., Keane, G., Bending, G. D., Chandler, D., Stobart, R., et al. (2013). Impact of shortened crop rotation of oilseed rape on soil and rhizosphere microbial diversity in relation to yield decline. PLoS ONE 8:e59859. doi: 10.1371/journal.pone.0059859
Hilton, S., Picot, E., Schreiter, S., Bass, D., Norman, K., Oliver, A. E., et al. (2021). Identification of microbial signatures linked to oilseed rape yield decline at the landscape scale. Microbiome 9:19. doi: 10.1186/s40168-020-00972-0
Hunter, P. J., Teakle, G. R., and Bending, G. D. (2014). Root traits and microbial community interactions in relation to phosphorus availability and acquisition, with particular reference to Brassica. Front. Plant Sci. 5:27. doi: 10.3389/fpls.2014.00027
Jones, D. L., Hodge, A., and Kuzyakov, Y. (2004). Plant and mycorrhizal regulation of rhizodeposition. New Phytol. 163, 459–480. doi: 10.1111/j.1469-8137.2004.01130.x
Kastl, E., Schloter-Hai, B., Buegger, F., and Schloter, M. (2014) “Impact of fertilization on the abundance of nitrifiers and denitrifiers at the root–soil interface of plants with different uptake strategies for nitrogen. Biol. Fertil. Soils 51, 57–64. doi: 10.1007/s00374-014-0948-1
Koljalg, U., Nilsson, R. H., Abarenkov, K., Tedersoo, L., Taylor, A. F. S., Bahram, M., et al. (2013). Towards a unified paradigm for sequence-based identification of fungi. Mol. Ecol. 22, 5271–5277. doi: 10.1111/mec.12481
Larkin, N. J., Ma, L., and Borhan, M. H. (2015). The Brassica napus receptor-like protein RLM2 is encoded by a second allele of the Lep3/Rlm2 blackleg resistance locus. Plant Biotechnol. J. 13, 983–992. doi: 10.1111/pbi.12341
Liang, S., Kou, X., Li, Y., Lü, X., Wang, J., and Li, Q. (2020). Soil nematode community composition and stability under different nitrogen additions in a semiarid grassland. Global Ecol. Conserv. 22:e00965. doi: 10.1016/j.gecco.2020.e00965
Love, M. I., Huber, W., and Anders, S. (2014). Moderated estimation of fold change and dispersion for RNA-seq data with DESeq2. Genome Biol. 15:550. doi: 10.1186/s13059-014-0550-8
Mahoney, A. K., Yin, C., and Hulbert, S. H. (2017). Community structure, species variation, and potential functions of rhizosphere-associated bacteria of different winter wheat (Triticum aestivum) Cultivars. Front. Plant Sci. 8:132. doi: 10.3389/fpls.2017.00132
Marschner, P., Solaiman, Z., and Rengel, Z. (2007). Brassica genotypes differ in growth, phosphorus uptake and rhizosphere properties under P-limiting conditions. Soil Biol. Biochem. 39, 87–98. doi: 10.1016/j.soilbio.2006.06.014
McDonald, D., Price, M. N., Goodrich, J., Nawrocki, E. P., DeSantis, T. Z., Probst, A., et al. (2012). An improved Greengenes taxonomy with explicit ranks for ecological and evolutionary analyses of bacteria and archaea. ISME J. 6, 610–618. doi: 10.1038/ismej.2011.139
Monreal, C., Zhang, J., Koziel, S., Vidmar, J., González, M., Matus, F., et al. (2018). Bacterial community structure associated with the addition of nitrogen and the dynamics of soluble carbon in the rhizosphere of canola (Brassica napus) grown in a Podzol. Rhizosphere 5, 16–25. doi: 10.1016/j.rhisph.2017.11.004
Morgan, J. A. W., Bending, G. D., and White, P. J. (2005). Biological costs and benefits to plant–microbe interactions in the rhizosphere. J. Exp. Bot. 56, 1729–1739. doi: 10.1093/jxb/eri205
Mur, L. A. J., Simpson, C., Kumari, A., Gupta, A. K., and Gupta, K. J. (2016). Moving nitrogen to the centre of plant defence against pathogens. Ann. Bot. 119, 703–709. doi: 10.1093/aob/mcw179
Oksanen, J., Blanchet, F. G., Friendly, M., Kindt, R., Legendre, P., McGlinn, D., et al. (2018). Vegan: Community Ecology Package. In {R package version 25-3}. Available online at: https://CRAN.R-project.org/package=vegan;
Paungfoo-Lonhienne, C., Yeoh, Y. K., Kasinadhuni, N. R. P., Lonhienne, T. G. A., Robinson, N., Hugenholtz, P., et al. (2015). Nitrogen fertilizer dose alters fungal communities in sugarcane soil and rhizosphere. Sci. Rep. 5:8678. doi: 10.1038/srep08678
Quast, C., Pruesse, E., Yilmaz, P., Gerken, J., Schweer, T., Yarza, P., et al. (2013). The SILVA ribosomal RNA gene database project: improved data processing and web-based tools. Nucleic Acids Res. 41, D590–D596. doi: 10.1093/nar/gks1219
Raman, H., Raman, R., Diffey, S., Qiu, Y., McVittie, B., Barbulescu, D. M., et al (2018) Stable quantitative resistance loci to blackleg disease in canola (Brassica napus L.) over continents. Front. Plant Sci. 9:1622. doi: 10.3389/fpls.2018.01622
Rathore, R., Dowling, D. N., Forristal, P. D., Spink, J., Cotter, P. D., Bulgarelli, D., et al. (2017). Crop establishment practices are a driver of the plant microbiota in winter oilseed rape (Brassica napus). Front. Microbiol. 8:1489. doi: 10.3389/fmicb.2017.01489
Revillini, D., Wilson, G. W. T., Miller, R. M., Lancione, R., and Johnson, N. C. (2019). Plant diversity and fertilizer management shape the belowground microbiome of native grass bioenergy feedstocks. Front. Plant Sci. 10:1018. doi: 10.3389/fpls.2019.01018
Selosse, M. A., Vohnik, M., and Chauvet, E. (2008). Out of the rivers: are some aquatic hyphomycetes plant endophytes? New Phytol. 178, 3–7. doi: 10.1111/j.1469-8137.2008.02390.x
Semenov, M. V., Krasnov, G. S., Semenov, V. M., and van Bruggen, A. H. (2020). Long-term fertilization rather than plant species shapes rhizosphere and bulk soil prokaryotic communities in agroecosystems. Appl. Soil Ecol. 154:103641. doi: 10.1016/j.apsoil.2020.103641
Sprague, S. J., Watt, M., Kirkegaard, J. A., and Howlett, B. J. (2007). Pathways of infection of Brassica napus roots by Leptosphaeria maculans. New Phytol. 176, 211–222. doi: 10.1111/j.1469-8137.2007.02156.x
Stoeck, T., Bass, D., Nebel, M., Christen, R., Jones, M. D. M., Breiner, H.-W., et al. (2010). Multiple marker parallel tag environmental DNA sequencing reveals a highly complex eukaryotic community in marine anoxic water. Mol. Ecol. 19, 21–31. doi: 10.1111/j.1365-294X.2009.04480.x
Tabrett, A., and Horton, M. W. (2020). The influence of host genetics on the microbiome. F1000Research 9:84. doi: 10.12688/f1000research.20835.1
Taye, Z. M., Helgason, B. L., Bell, J. K., Norris, C. E., Vail, S., Robinson, S. J., et al. (2020). Core and differentially abundant bacterial taxa in the rhizosphere of field grown brassica napus genotypes: implications for canola breeding. Front. Microbiol. 10:3007. doi: 10.3389/fmicb.2019.03007
Wagner, M. R., Lundberg, D. S., del Rio, T. G., Tringe, S. G., Dangl, J. L., and Mitchell-Olds, T. (2016). Host genotype and age shape the leaf and root microbiomes of a wild perennial plant. Nat. Commun. 7:12151. doi: 10.1038/ncomms12151
Wang, H., Gu, C., Liu, X., Yang, C., Li, W., and Wang, S. (2020). Impact of soybean nodulation phenotypes and nitrogen fertilizer levels on the rhizosphere bacterial community. Front. Microbiol. 11:750. doi: 10.3389/fmicb.2020.00750
Wang, J., Xue, C., Song, Y., Wang, L., Huang, Q., and Shen, Q. (2016). Wheat and rice growth stages and fertilization regimes alter soil bacterial community structure, but not diversity. Front. Microbiol. 7:1207. doi: 10.3389/fmicb.2016.01207
Wei, F., Zhao, L., Xu, X., Feng, H., Shi, Y., Deakin, G., et al. (2019). Cultivar-dependent variation of the cotton rhizosphere and endosphere microbiome under field conditions. Front. Plant Sci. 10:1659. doi: 10.3389/fpls.2019.01659
Whipps, J. M. (2001). Microbial interactions and biocontrol in the rhizosphere. J. Exp. Bot. 52, 487–511. doi: 10.1093/jxb/52.suppl_1.487
White, T. J., Bruns, T., Lee, S., and Taylor, J. (1990). PCR Protocols: A Guide to Methods and Applications. New York, NY: Academic Press.
Zhong, Y., Yang, Y., Liu, P., Xu, R., Rensing, C., Fu, X., et al. (2019). Genotype and rhizobium inoculation modulate the assembly of soybean rhizobacterial communities. Plant Cell Environ. 42, 2028–2044. doi: 10.1111/pce.13519.
Keywords: rhizosphere, crop genotype, nitrogen, protist, nematode, fungi, pathogens, interaction networks
Citation: Picot E, Hale CC, Hilton S, Teakle G, Schäfer H, Huang Y-J, Perryman S, West JS and Bending GD (2021) Contrasting Responses of Rhizosphere Bacterial, Fungal, Protist, and Nematode Communities to Nitrogen Fertilization and Crop Genotype in Field Grown Oilseed Rape (Brassica napus). Front. Sustain. Food Syst. 5:613269. doi: 10.3389/fsufs.2021.613269
Received: 01 October 2020; Accepted: 04 March 2021;
Published: 08 April 2021.
Edited by:
Adam C. N. Wong, University of Florida, United StatesReviewed by:
Blanca B. Landa, Spanish National Research Council, SpainWu Xiong, Utrecht University, Netherlands
Copyright © 2021 Picot, Hale, Hilton, Teakle, Schäfer, Huang, Perryman, West and Bending. This is an open-access article distributed under the terms of the Creative Commons Attribution License (CC BY). The use, distribution or reproduction in other forums is permitted, provided the original author(s) and the copyright owner(s) are credited and that the original publication in this journal is cited, in accordance with accepted academic practice. No use, distribution or reproduction is permitted which does not comply with these terms.
*Correspondence: Gary D. Bending, gary.bending@warwick.ac.uk